
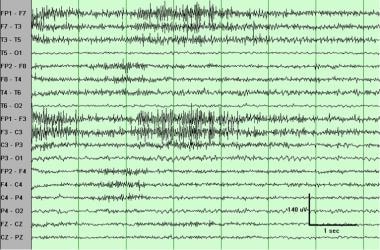
In most cases, ICA methods yield a useful separation, but some mixed components remain which contain activity from both artifactual and neural origin (see, e.g., for a review of the current state-of-the-art). The most commonly deployed techniques to achieve this goal are variants of independent component analysis (ICA), which solve the blind source separation (BSS) problem by maximizing the independence of the source components. The overall goal is to separate artifactual from neural activity in different components, such that the artifactual components can be discarded and cleaner signals can be reconstructed from the neural components only. The most widespread technique to reduce muscle artifacts in EEG recordings is the linear decomposition of EEG signals into source components. Because muscle activity arises from different type of muscle groups, muscle artifacts are harder to stereotype than eye artifacts (cf. These are typically caused by muscle activity near the head, such as swallowing or head movements, and are characterized by high-frequency activity (> 20 Hz). In this paper, we are concerned with the removal of muscle artifacts. Such artifacts stem from eye and muscle movement, the heart beat or external technical sources. The removal of undesired artifacts from the electroencephalogram (EEG) is a major preprocessing step for most EEG analysis.
